In today’s digital age, movie recommendations play a crucial role in guiding viewers towards the perfect film choice that aligns with their taste and preferences. With the wide array of movies available across various platforms, finding the ideal movie can often feel like searching for a needle in a haystack. However, through the amalgamation of advanced algorithms, user data analysis, and personalized suggestions, the quest for the perfect movie recommendation has never been more promising.
One of the key ingredients in the recipe for a successful movie recommendation system is the utilization of advanced algorithms such as collaborative filtering and content-based filtering. Collaborative filtering, a method often employed by streaming platforms like Netflix and Hulu, analyzes user behavior and preferences to generate recommendations based on the viewing history and ratings of similar users. This technique effectively suggests movies that align with the tastes of the viewer, increasing the likelihood of a successful movie-watching experience.
On the other hand, content-based filtering focuses on the attributes of the movies themselves, such as genre, director, actors, and plot keywords. By identifying commonalities between movies that a viewer has enjoyed in the past and leveraging machine learning algorithms to recommend similar content, platforms can enhance the accuracy of their movie recommendations. This personalized approach to suggesting films ensures that viewers are introduced to new and exciting content that resonates with their cinematic inclinations.
Moreover, the collection and analysis of user data play a pivotal role in the efficacy of movie recommendation systems. By tracking viewing habits, genre preferences, ratings, and even the time of day a viewer typically watches movies, platforms can glean valuable insights into individual preferences. Leveraging this data allows recommendation algorithms to tailor suggestions to the unique tastes of each user, ultimately enhancing the overall viewing experience.
In addition to algorithmic sophistication and data analysis, the evolution of movie recommendation systems has seen a rise in the popularity of hybrid recommendation approaches. By combining collaborative filtering and content-based filtering techniques, platforms can offer a more comprehensive and accurate movie recommendation service. This hybrid model harnesses the strengths of both methods, leveraging user behavior and movie attributes to deliver personalized suggestions that cater to the diverse preferences of viewers.
In conclusion, the pursuit of the perfect movie recommendation has been significantly enhanced by the advancements in algorithmic development, user data analysis, and personalized suggestion techniques. By harnessing the power of collaborative filtering, content-based filtering, and hybrid recommendation approaches, platforms can offer viewers a curated selection of movies that align with their individual tastes and preferences. With the cinematic landscape expanding and diversifying, the importance of accurate and tailored movie recommendations has never been more pronounced, ushering in a new era of personalized entertainment experiences for audiences worldwide.
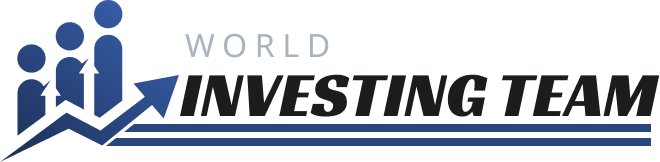