In the ever-evolving landscape of artificial intelligence (AI) research and development, one issue that has begun to surface with increasing frequency is the phenomenon of AI hallucinations. These hallucinations, in which AI systems interpret sensory information incorrectly and generate false outputs, introduce a significant challenge to the reliability and safety of AI technologies.
To understand the implications of AI hallucinations, it is crucial to delve into how these phenomena occur and the potential risks they pose. At the core of AI hallucinations lies the concept of neural network architectures, which serve as the building blocks of many AI systems. Neural networks consist of interconnected nodes that process and analyze data to make predictions or decisions. Through a series of training iterations, neural networks learn to recognize patterns and correlations within datasets, enabling them to perform complex tasks such as image recognition or language processing.
However, despite their remarkable capabilities, neural networks are not infallible. AI hallucinations can occur when a neural network misinterprets patterns in the data it is processing, leading to the generation of erroneous outputs. These outputs can manifest in various forms, such as generating distorted images, mislabeling objects, or producing nonsensical text. In some cases, AI hallucinations can go unnoticed during the development and testing phases of AI systems, only to surface unexpectedly in real-world applications.
The implications of AI hallucinations are far-reaching, encompassing both ethical and practical considerations. From an ethical standpoint, the presence of hallucinations in AI systems raises concerns about the potential impact on decision-making processes. If AI systems are prone to generating false outputs, there is a risk that critical decisions based on these outputs could have detrimental consequences. For example, in autonomous vehicles, a hallucination that misidentifies a stop sign could result in a serious accident.
Moreover, the practical implications of AI hallucinations are equally significant. In sectors where AI technologies are increasingly being integrated, such as healthcare, finance, and transportation, the accuracy and reliability of AI systems are paramount. The presence of hallucinations undermines the trustworthiness of these systems, creating barriers to their widespread adoption and deployment.
Addressing the issue of AI hallucinations requires a multi-faceted approach that encompasses both technical solutions and ethical frameworks. One key strategy is to enhance the interpretability of neural networks, enabling researchers and developers to gain deeper insights into how these systems arrive at their decisions. By enhancing transparency and traceability in AI models, stakeholders can mitigate the risks associated with hallucinations and improve the overall reliability of AI technologies.
Furthermore, ethical guidelines and regulations play a crucial role in guiding the responsible development and deployment of AI systems. Establishing clear standards for AI testing, validation, and monitoring can help prevent the emergence of hallucinations and ensure that AI technologies are deployed in a safe and ethical manner. Additionally, fostering collaboration between researchers, industry stakeholders, and policymakers is essential to address the complex challenges posed by AI hallucinations.
In conclusion, the issue of AI hallucinations represents a pressing concern that requires urgent attention from the AI research community. By understanding the underlying causes of hallucinations, implementing technical safeguards, and establishing ethical guidelines, we can work towards harnessing the full potential of AI technologies while mitigating the risks associated with hallucinations. Only through proactive and collaborative efforts can we ensure that AI systems remain trustworthy, reliable, and safe in an increasingly AI-driven world.
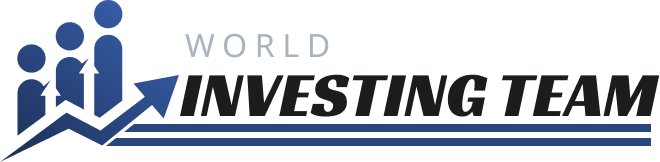